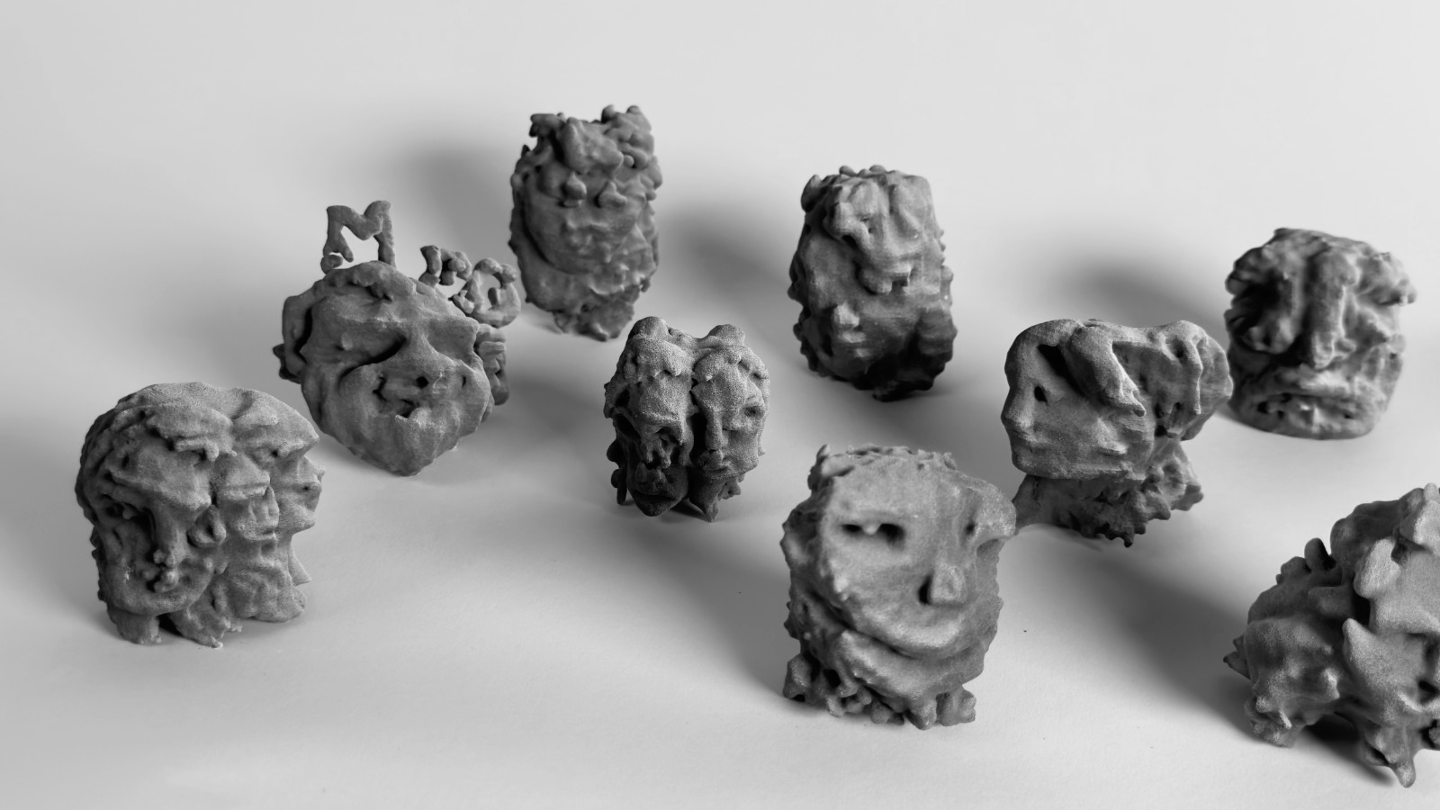
Gender Glitch Matrix: Non-binary Objects
Machine Learning | Sculpture
as part of the MIT Architecture SMArchS Computation thesis:
"Gender Glitch Matrix: Queer Aesthetics and the Politics of Error in Digital Media"
Project: Merve Akdogan
Advisors: Azra Akšamija, Larry Sass
Reader: Panagiotis Michalatos
Time: May 2024
This experiment involves a series of machine learning-based attribution tests focusing on gender bias. I attempted to identify non-binary in-between genders by experimenting with different prompts matching the model's binary codes and combining gender attribution with these keywords. This study brought to light vital shortcomings in existing AI technologies, such as stereotypical attribution assigned to gender, which causes the model to "glitch" when interpreting gender when binary expressions are mixed. This study offers a critical analysis of the binary restrictions of gender as they are frequently represented in technology and compels us to consider how AI both supports and subverts social norms. The following research should concentrate on refining these techniques, expanding the vocabulary of gender and queer terms in AI datasets, and exploring more complex models that better capture and represent the nuances of gender identity. This last experiment shows how comprehensive glitches can be in challenging and subverting AI systems' encoded biases, which connects to earlier experiments.
In this experiment, the media is the machine learning model itself, and the purpose is to understand how this model understands binary gender representations. Using differential volumetric rendering, I aimed to explore the biases in CLIP's perception of gender by manipulating prompts that generate 3D models. By doing so, we can reproduce the machine's three-dimensional perception, revealing its inherent biases and how they shape the output.
The project uses the popular machine learning model, CLIP, from OpenAI. In keeping with the evaluation of the CLIP model, the manipulation of 3D volume representations through optimization techniques was mainly focused on assessing gender bias by testing various prompts associated with gender terminology. The gender interpretation of the CLIP model represents this instance’s “glitch” since the model’s fundamental error is that the binary gender has highly normative and stereotypical ideas. The method uses a differentiable volumetric rendering system and a loss function that the CLIP model guides. The volumetric density and color fields are rendered from various angles, and the optimizer tries to find the voxel density distribution that maximizes similarity to the clip prompt. Simply, the system processes the volume by illuminating each slice with a volumetric renderer. It then attempts to make the model similar to the prompt by optimizing a loss function.
This method relies on adjusting model parameters to minimize a composite loss function. The optimization dynamically changes the volume representation to satisfy conditions such as similarity to specific texts (for instance, “feminine” positively and “masculine” negatively influenced), which are processed through the CLIP model to generate relevant semantic loss. The optimization actively tries to find something between “feminine” and “masculine” that reflects the criticism of fluidity and performativity of gender.
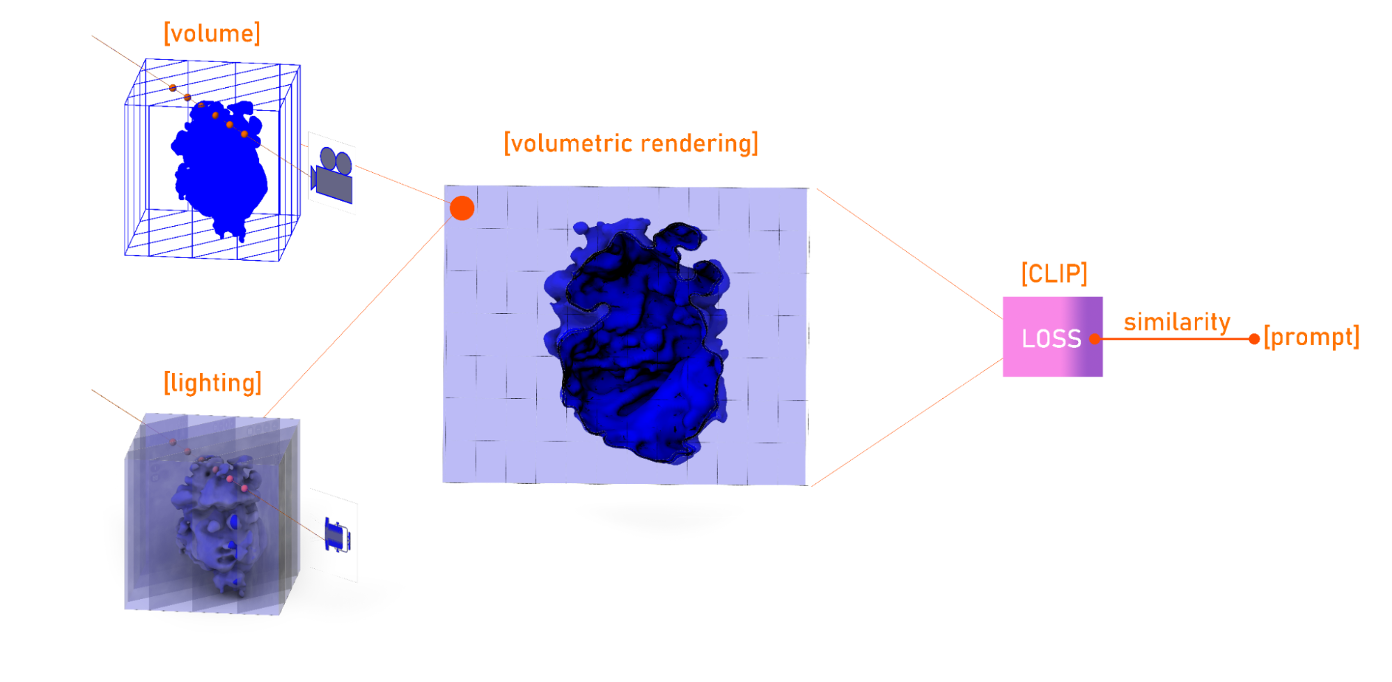
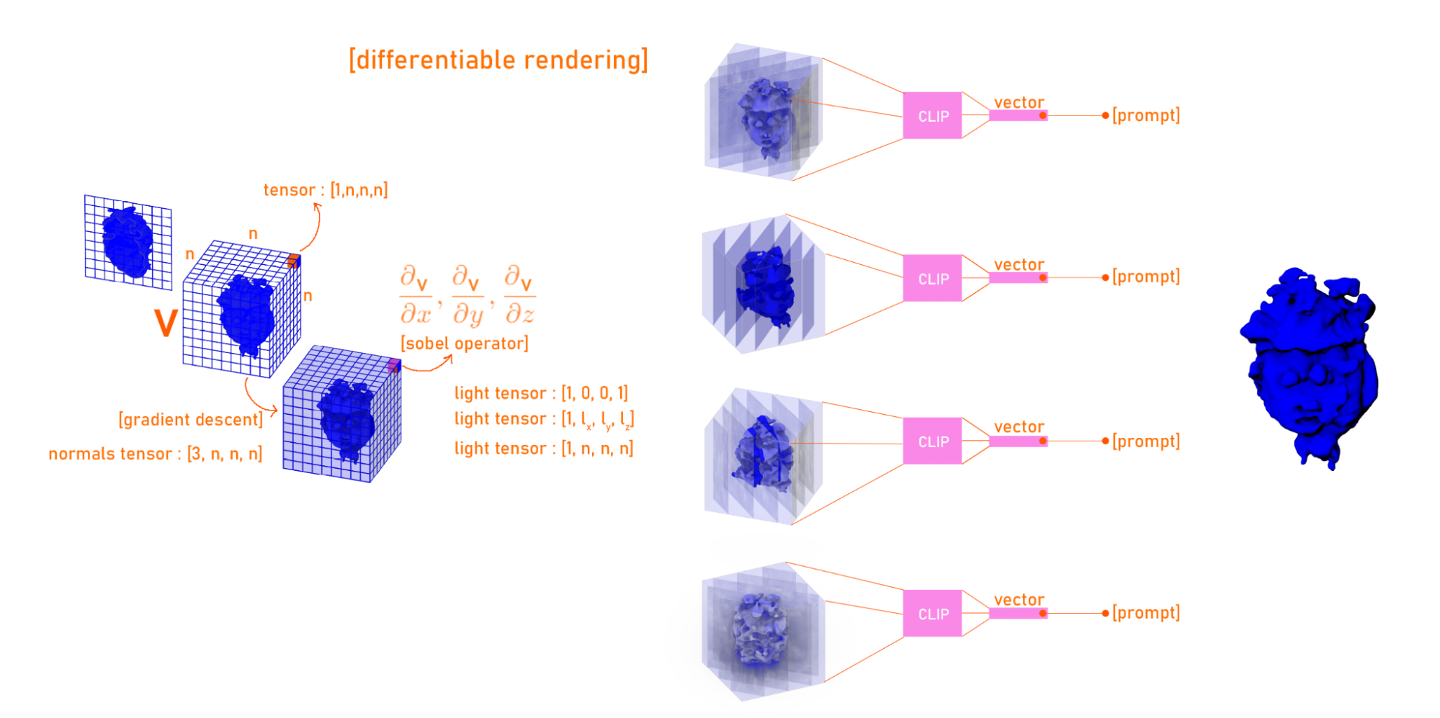
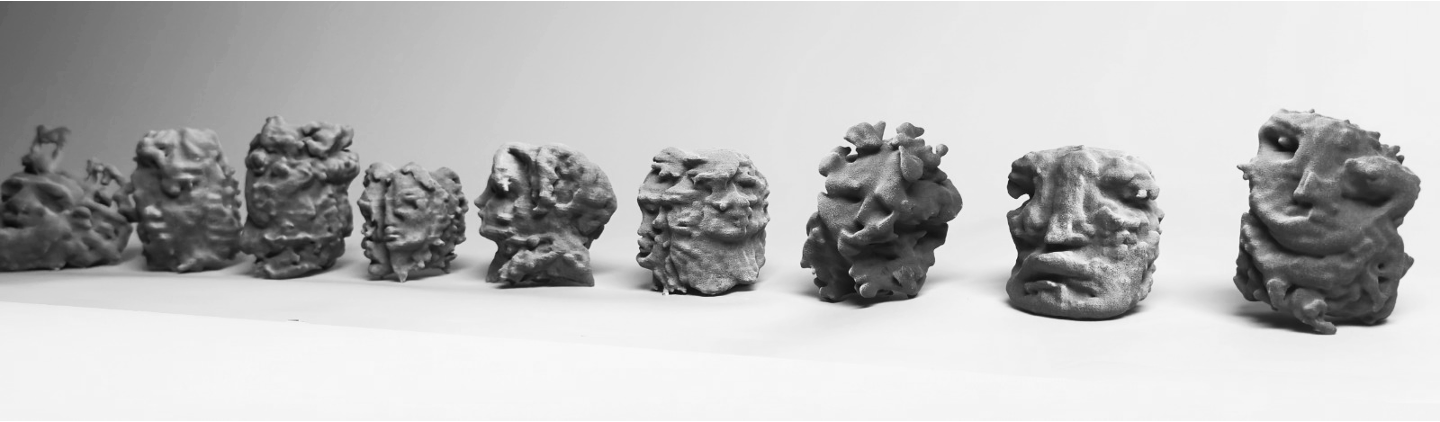

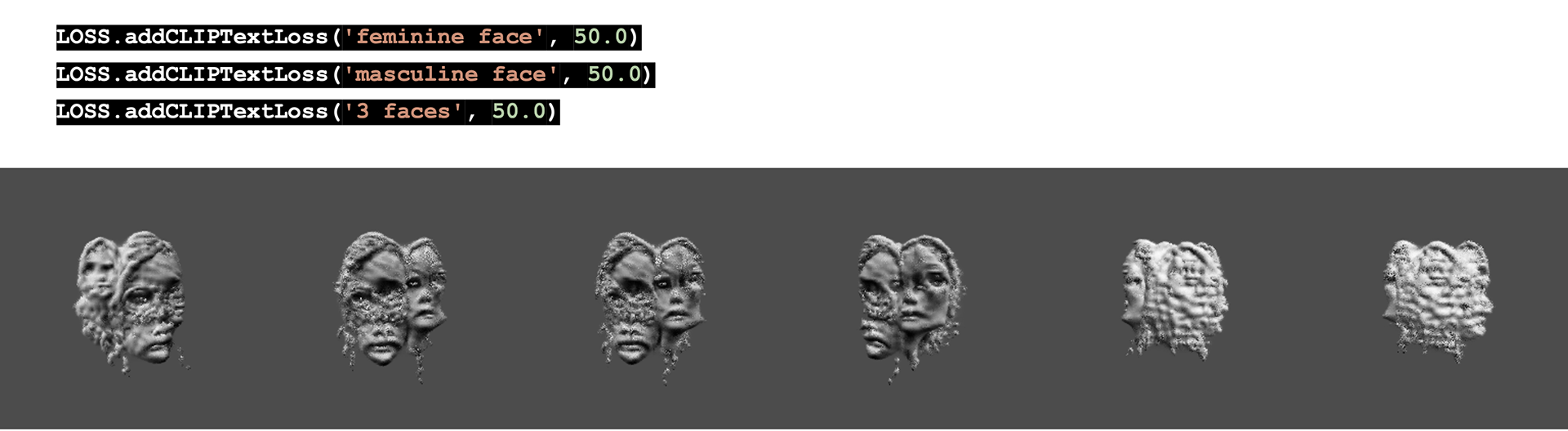
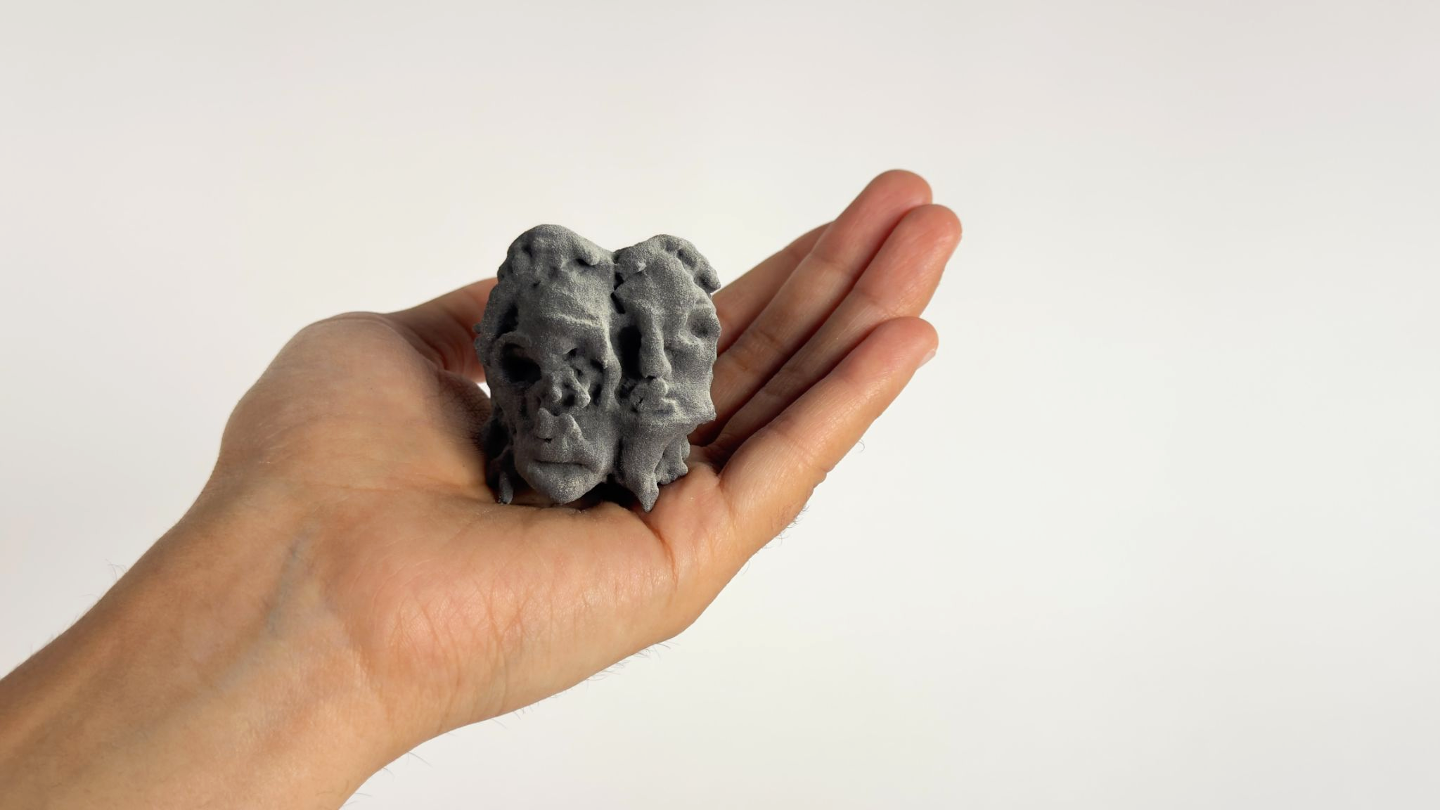
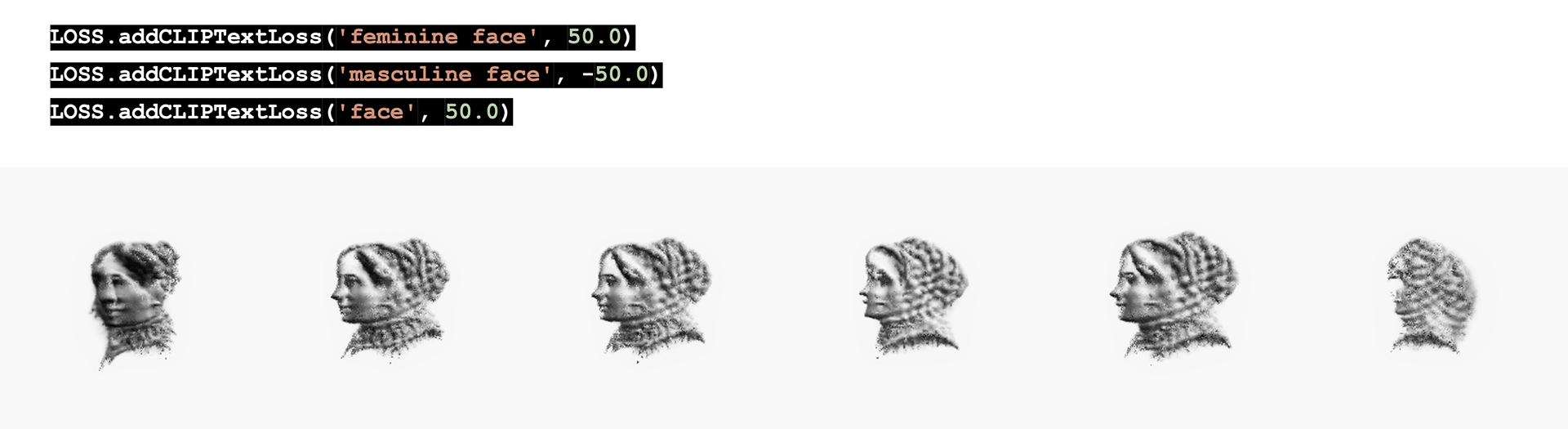
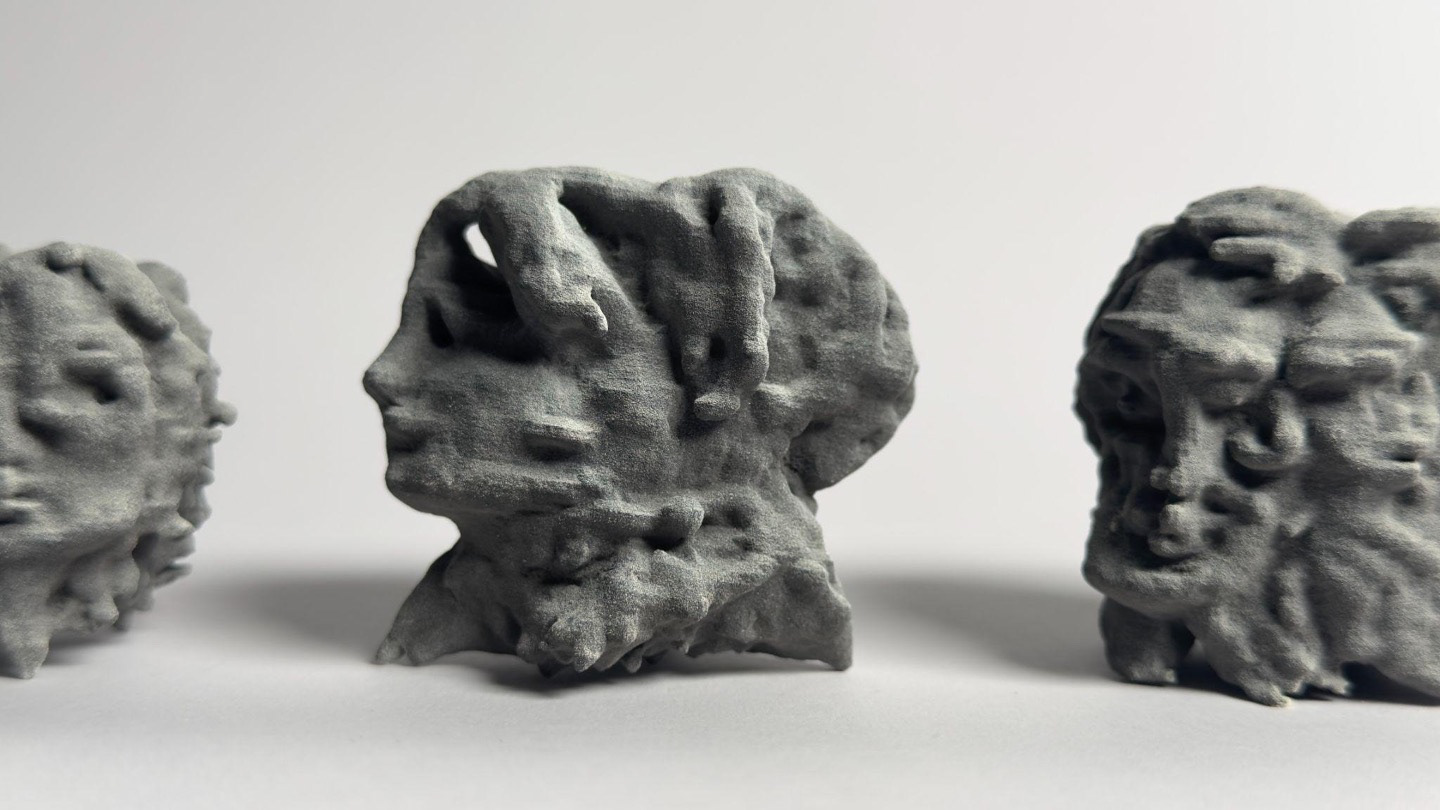
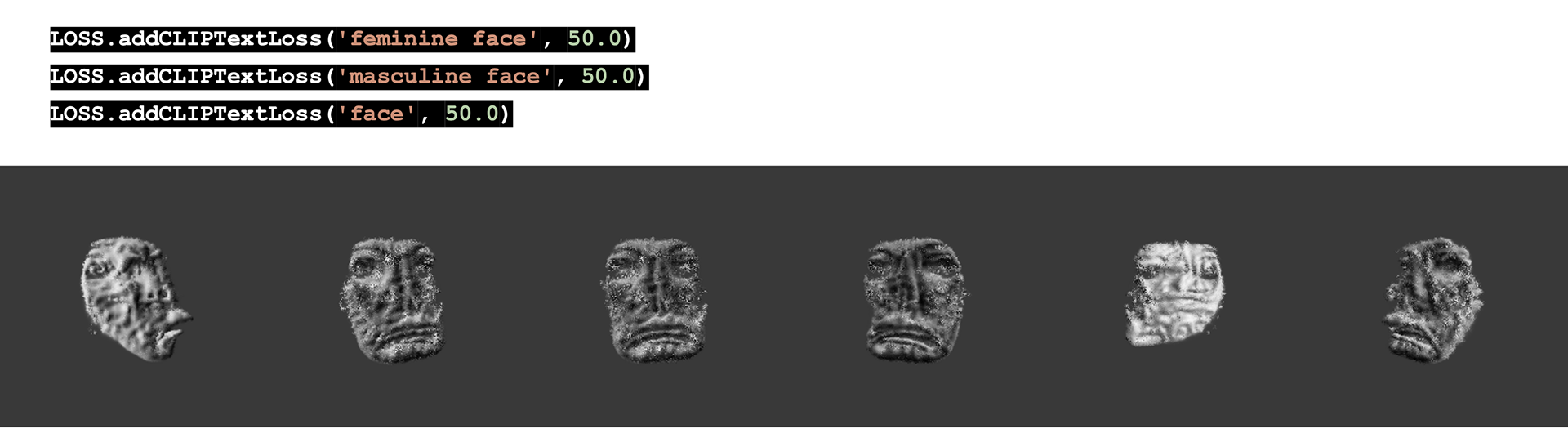
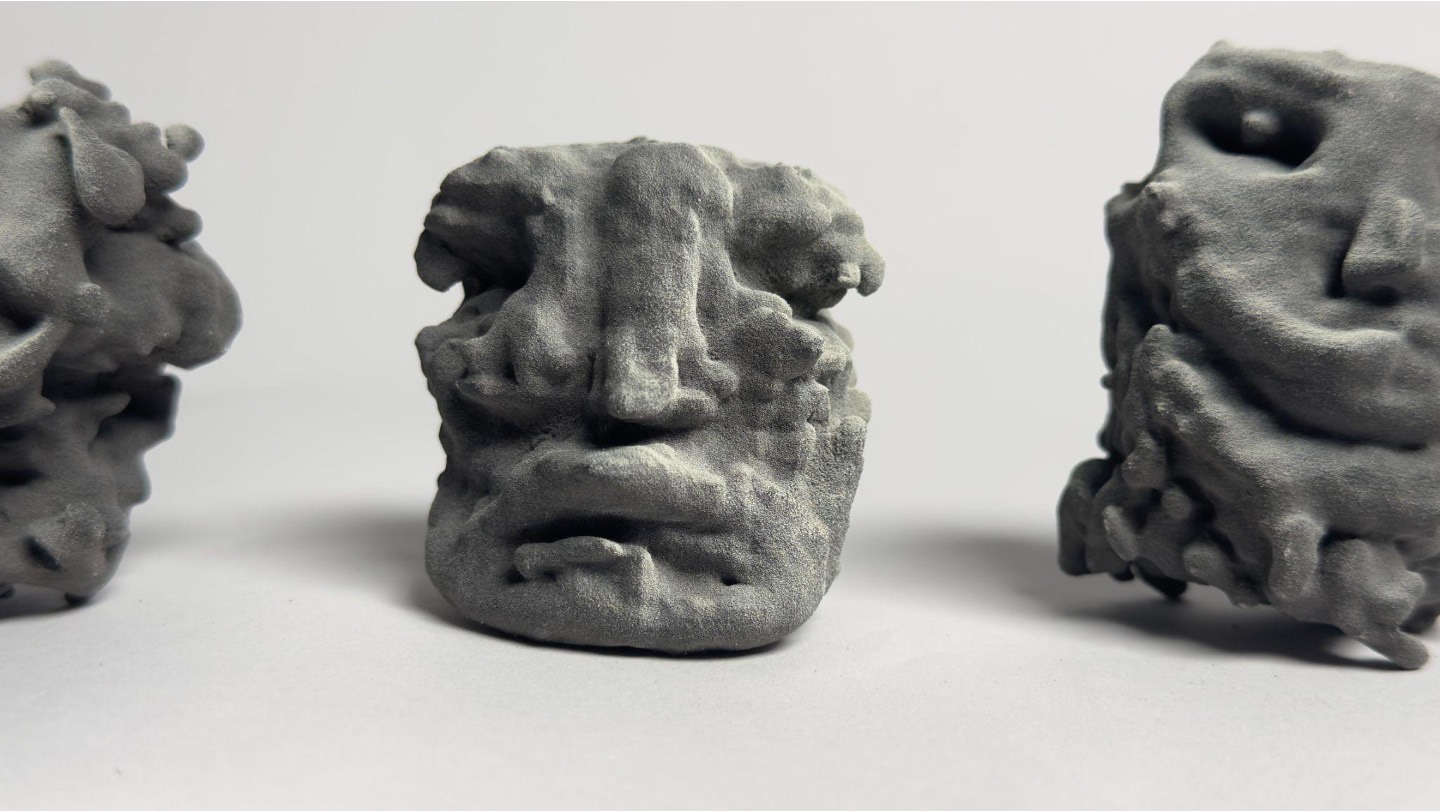
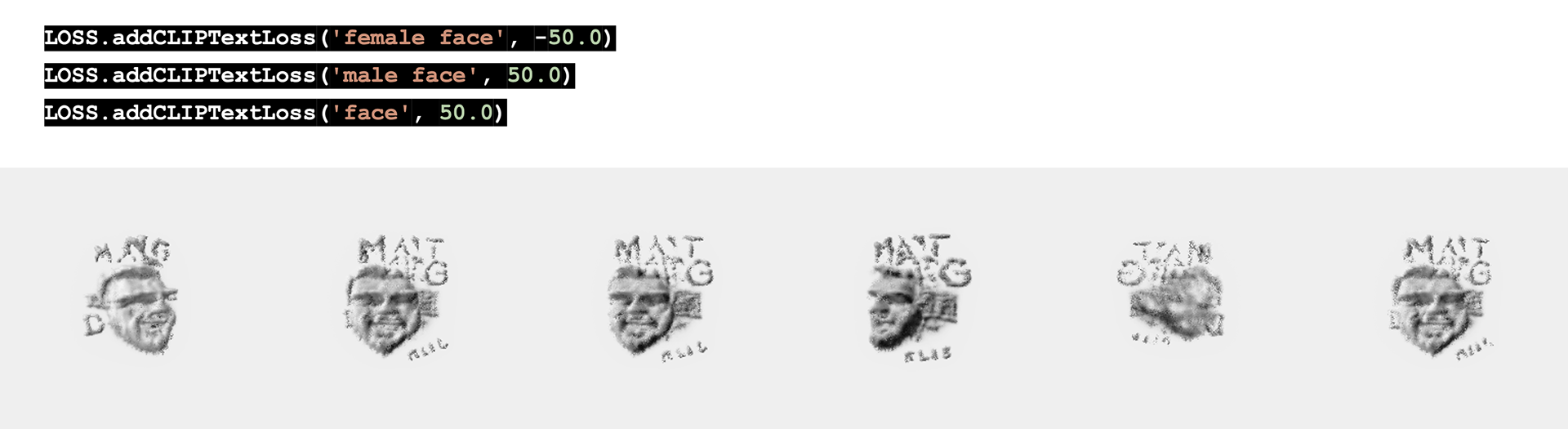
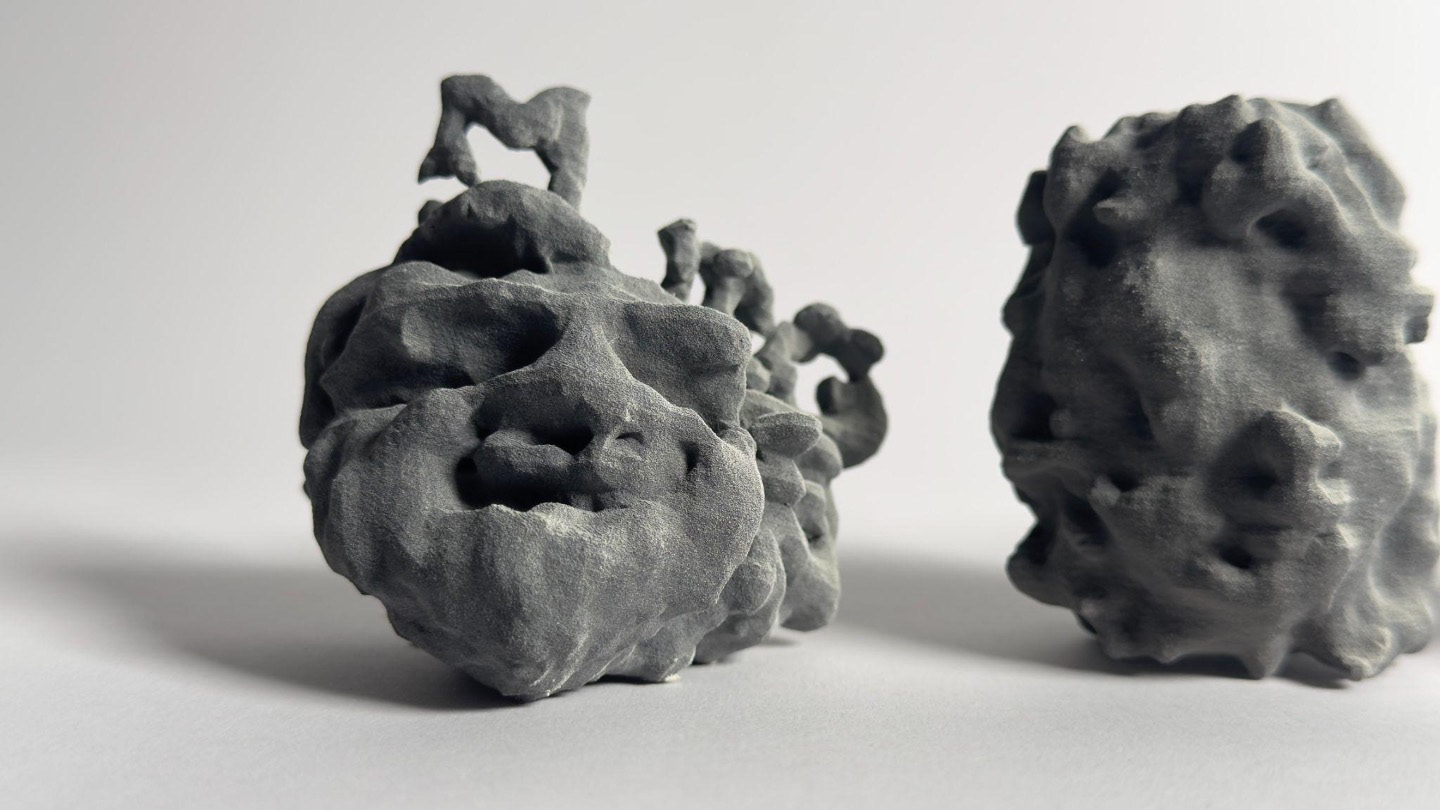